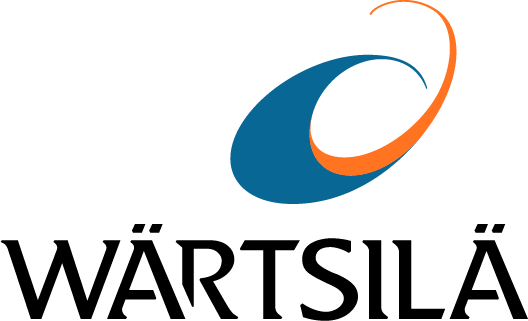
What do you see when you think of seafarers onboard and onshore using data from a ship? Is it someone standing at a terminal looking at analytics on a dashboard, deeply engrossed in the decision-making process?
The maritime industry
has come a long way in its use of big data in the past decade. Digital technologies and advanced analytics platforms are giving seafarers enhanced operational insights and support in decision-making processes like never before.
As the availability of data for high performance computing is increasing alongside the adoption of automation, Markus Mannevaara, Senior Director, Rapid Innovation at Wärtsilä Voyage, believes that artificial intelligence and machine learning
are technologies to keep an eye on.
“The industry is on a large data-driven journey, and soon it will have a machine learning ‘ah ha’ moment down the line,” he says.
As the lead of the Rapid Innovation team – the people at Wärtsilä
Voyage who turn breakthrough technologies into useful ship solutions – Mannevaara has helped develop the latest intelligent bridge systems and the user experience framework being deployed across Wärtsilä Voyage’s systems. His
background is in software development and when asked about data, people, and the shipping industry, his thoughts head straight to the long-term future, to machine learning and its potential in the industry. He has a keen eye for digital markets and
takes lessons from the uses of machine learning in other industries.
“In maritime, I find that we tend to have a mechanical, almost simplistic notion of how the availability of data will change behaviour,” says Mannevaara.
“While ship owners grasp the benefits of digitalisation, there is much further to go in shipping’s transformation,” he adds.
Estimates show that 90% of data generated onboard the ship never leaves the deck,
which means operators lose invaluable insight and analytics that can improve performance and efficiency every day. This data and digitally connected operations are key to unlocking ship efficiency, particularly when the industry is so focused on decarbonisation.
However, unlocking the power of data and ensuring that it is used in the most optimal way can be tricky if the right technologies are not in place. Seafarers today have enough on their daily task lists, and any system introduced onboard must alleviate
workload and not add burden.
Digital technology is ultimately an enabler of problem solving for people onboard and onshore. Taking problem solving from traditional methods to automated methods is where Mannevaara sees most potential for making the day-to-day work of seafarers easier.
Currently, leveraging data in decision making and operations automation in the maritime industry still requires human input or a human decision point, but imagine that human time requirement being removed and replaced. That is enabled by machine learning,
a branch of AI that focuses on the use of data and algorithms to imitate the way that humans learn, gradually improving its accuracy.
However, for machine learning use, better data mining is required. While data availability
and fidelity in the maritime industry is improving, it’s a long way off machine learning standards. Consequently, this has stifled the application of machine learning techniques in maritime transport compared to other industries, where AI helps
detecting financial frauds and diagnosing cancer more accurately.
What we are talking about here is analogous to control loops in automation systems. But the maritime industry isn’t using data in a widespread way, yet. The industry
that moves 90% of the world’s goods is taking a traditional approach to analytics. It may not be as mature as financial services or healthcare, as highlighted in the examples above, but that doesn’t mean this isn’t somewhere the
industry can be in the future.
Some areas of the maritime industry are already starting to engage with machine learning. For example, Brittany Ferries decided to use Wärtsilä Voyage’s energy management and optimisation technologies to increase fuel efficiency and reduce emissions. For Livestock Express, a Vroon company and an operator of a fleet of 15 livestock carriers, the installation of Wärtsilä Voyage’s Fleet Optimisation Solution (FOS) has not only enabled crews to optimise safe and smooth navigation; the added visibility of a dedicated ship-to-shore connection for navigation has allowed
the company to increase remote support for crews, report daily conditions to regulators and improve training for shoreside operators.
However, Mannevaara believes the place where machine learning could have the most profound impact is in shipping and logistics. The decision whether to charter vessel A or vessel B is currently a manual process. This is where machine learning would be
most useful, he believes, as the process would be so much faster if human involvement was removed.
“Chartering operations is the area of the industry that stands to gain the most from machine learning adoption in the shortest
timeframe,” he says.
When it comes to real-time decision processes on board a ship, automation systems are already in use with great success, although this is another area that could benefit from closed loop machine learning.
But what is closed loop machine learning, we hear you ask? It is the creation of a cycle between the intelligence of a system and the usage of the system. As the intelligence improves, users get more benefits from the system and as more users
engage with the system, they generate more data to improve the intelligence.
On board a ship this could take the form of automatic execution of speed and route profiling. Savings could be achieved if that were to happen en masse,
with all ships executing speed and voyage profiles automatically. Another area on board is to control the power plant in an optimal way, since manual operations are the norm here. A computer would be able to decide between having two engines running
at 60% load versus one at 80% load.
But for now, achieving greater levels of automation, with everything in the automated system being manually calibrated and programmed, is how it will be for the foreseeable future.
Turning his thoughts to where the trail of closed
loop machine learning could be best placed, Mannevaara believes that closed loop voyage optimisation is totally do-able with today’s technology. It can be where savings are productively achieved, but he also says that what takes time is making
sure these closed loop systems are safe.
The industry may be a way off adopting machine learning just yet, but it is possible to put machine learning as a layer on top of other existing data-driven processes. What is more profound is the emergent behaviour when you have data available and use it to improve application or user experience.
- Markus Mannevaara, Senior Director, Rapid Innovation, Wärtsilä Voyage
Technology, that has been advancing by leaps and bounds, has always supported seasoned mariners in their pursuit of making the oceans safer and easier to navigate. Digitalisation and cloud-tech are doing so at an unprecedented pace. The true power
of today’s software algorithms is that they make recommendations that can then be analysed and interpreted by the user to course-correct, blending software insights with human reasoning to chart the best course. The chances of human error go
down, data quality goes up, plus the crew has more time to concentrate on critical decision-making — a much better utilisation of their skill sets and time.
Technology is there, and it has a lot of potential. Now let us figure out how you can safely achieve even greater goals.
- Markus Mannevaara, Senior Director, Rapid Innovation at Wärtsilä Voyage