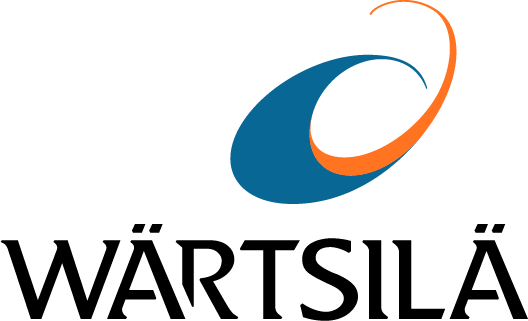
In the asset management domain, systems designed to detect anomalies and potential failures are referred to as condition monitoring, or, CM systems. Some CM systems use specific techniques such as thermography and vibration analysis for rotating equipment. These techniques are effective, but their applicability is limited to specific failure modes. By far, however, most CM systems rely on rules based on engineering knowledge. Both rules and the engines that evaluate them have been improved incrementally over the years to, for example, consider more parameters and conditions, or to be run more frequently. For every issue that needs to be detected an engineer needs to design a rule that can detect it.
As machinery becomes more complex, more issues may occur, and more rules are needed to monitor the system. The better a traditional CM system becomes at detecting issues the more numerous and complex the rules become. Eventually the system becomes difficult to maintain and, in some cases, unreliable. Furthermore, engineered rules are specific to one kind of machinery. Monitoring another kind of machinery entails creating a completely new set of rules.
Often actual failures occurring in the field trigger the creation of new- or better rules to detect those, previously unknown or misunderstood failure modes. Hence, in practice it can take years before a CM system to reach maturity; meaning it is able to detect critical failures.
For engines Wärtsilä has a good CBM (Condition Based Maintenance) service available. Its rules and formulas have been improved and refined for many years and it is able to detect many critical failure modes in a timely fashion. Nonetheless, like other systems that rely on rules, it takes considerable effort to maintain, and expanding the current approach to other equipment types would be cumbersome.
Fortunately, thanks to advancements in the field of artificial intelligence and technologies such as Google’s TensorFlow, we are now on the verge of a paradigm shift, where we move from:
Because the ML algorithms automatically analyse all incoming data in real-time, the equipment experts can focus fully on supporting the customer, rather than creating rules, crunching data and making reports. By combining raw processing power of an AI with the deep understanding of equipment experts we can create the CBM system of the future and support our customers much better.
Compared to a human being, a well-trained AI is superior at detecting anomalous behaviour. Whereas it might take a human being several days to go through a month of machinery data, with varying results, an AI might process it in less than a minute with reliable results.
Unfortunately, AIs today do not yet have the capability to interpret the anomalies. They cannot determine whether an anomaly is a precursor of a severe failure or a less problematic defect, which has no effect on machinery performance, such as a broken sensor. This is where the equipment expert comes in. With knowledge and experience in engineering and troubleshooting an equipment expert can review the anomaly and provide a recommendation to the operator.
If the anomaly detected by the AI indicates a valid issue, the Wärtsilä expert can notify the on-board crew the moment it occurs, thus preventing the situation from worsening.
It is also possible that the AI makes a mistake and finds an anomaly which is in fact normal equipment behaviour, or it can miss an event in its entirety. This is where one of the main strengths of machine learning comes in play: its ability to continue improving even when in active use. By pointing out the mistakes of the AI, the expert can teach the AI to correctly assess similar cases in the future.
When doing predictive maintenance on engines the learning capability of the AI shines. A challenge with combustion engines is that every engine is unique due to its environment, load characteristics, the way piping is mounted, and other factors. Whilst it might be too labour intensive to create a single rule set for each engine, it takes virtually no effort to train an AI for each engine. This makes it possible to fine-tune the algorithms for each engine, making it possible to handle engine specific differences without sacrificing accuracy.
A rule-based approach relies on engineering knowledge on how components within machinery interact. Creating an AI however is fundamentally different. When an AI is fed with sensory data it learns the relationship between measured signals. Whenever one of the signals deviates from what the AI has learned as normal, this is considered as anomalous and flagged as a potential issue.
Such a data driven approach has the advantage that the same method can easily be applied on different types of equipment. From combustion engines to HVAC systems, if it is equipped with sensors, an AI can be trained to detect anomalies with minimal configuration.
The traditional method of communication in CBM systems has been periodic reporting. This makes sense in a setup where the expert spends a lot of time on analysis, and addresses each installation one by one, typically in a monthly cycle. When however, the ML algorithms crunch the data, the expert can focus on diagnosing anomalies and providing recommendations to the customer.
In such a setup, the monthly reports no longer make sense. One would be tempted to resort to e-mails and phone calls, but such would ignore the fact that there are dozens or even hundreds of machineries on-board a ship or on a power plant. A better alternative is to use a collaboration application that allows cases to be reviewed in context.
An overview of the analysis software and the collaboration application are included on the overview below. After the equipment expert has diagnosed a potential issue and included its recommendations it becomes visible in the collaboration application. The expert then collaborates with the operator to resolve the issue. It’s a two-way street, and the operator can also request a 2nd opinion from the expert on issues experienced at the site.
Click on the image for a full view.
Wärtsilä has engaged in a small -scale pilot with Royal Caribbean Cruise Lines (RCCL). RCCL is a front-runner when it comes to the adoption of new digital technologies. Thus far the ML algorithms and the assigned experts have been able to detect all potential issues in a timely manner, allowing the crew on-board to take timely preventive- and optimisation actions.
Wärtsilä has a broad portfolio of equipment and experts supporting those equipment in the field. Leveraging AI- and machine learning technology allows our experts to focus on supporting our customers and to provide recommendations proactively in real-time.
These new technologies are anticipated to greatly enhance both the service level Wärtsilä customers experience, as well as the performance of equipment in the field. In addition, it opens the doors to new business models and revenue streams that were not possible before. The future is bright.
The term ‘artificial intelligence’ or AI dates back to 1956 and is attributed to John McCarthy, a Stanford researched working in the field of computer science. AI is the ability of a machine or a computer program to learn. The concept of AI is based on the idea of building machines capable of learning like humans and then be able to think and act on the basis of those learnings.
The terms machine learning and AI are often used interchangeable but they are not the same. AI is a broad concept while machine learning is a common application of AI in today’s industry. In machine learning (ML), algorithms are created that use computational methods to help the machine gather and therefore “learn” information directly from data without relying on pre-set logic, such as the rules created by an engineer. ML algorithms adaptively improve their performance as the number of samples available for learning increases.
The AI referenced in this article consists of several such ML algorithms. Typically, a ML system consists of three major parts: the model which makes the predictions, the parameters used by the model to weigh different inputs and the learner which adjusts the parameters based on the ability of the model to predict the right outcome. A simple overview is included below.
Author: Frank Velthuis, Director Digital Development, Digital Product Development, frank.velthuis@wartsila.com